A digital algorithm using 24 patient characteristics identifies far more women who are likely to develop a postpartum hemorrhage than currently used tools to predict the risk for bleeding after delivery, according to a study published in the Journal of the American Medical Informatics Association.
About 1 in 10 of the roughly 700 pregnancy-related deaths in the United States are caused by postpartum hemorrhage, according to the US Centers for Disease Control and Prevention. These deaths disproportionately occur among Black women, for whom studies show the risk of dying from a postpartum hemorrhage is fivefold greater than that of White women.
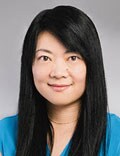
Dr Li Li
“Postpartum hemorrhage is a preventable medical emergency but remains the leading cause of maternal mortality globally,” the study’s senior author Li Li, MD, senior vice president of clinical informatics at Sema4, a company that uses artificial intelligence and machine learning to develop data-based clinical tools, told Medscape Medical News. “Early intervention is critical for reducing postpartum hemorrhage morbidity and mortality.”
Porous Predictors
Existing tools for risk prediction are not particularly effective, Li said. For example, the American College of Obstetricians and Gynecologists’ (ACOG) Safe Motherhood Initiative offers checklists of clinical characteristics to classify women as low, medium, or high risk. However, 40% of the women classified as low risk based on this type of tool experience a hemorrhage.
ACOG also recommends quantifying blood loss during delivery or immediately after to identify women who are hemorrhaging because imprecise estimates from clinicians may delay urgently needed care. Yet many hospitals have not implemented methods for measuring bleeding, said Li, who also is an assistant professor of genetics and genomic sciences at the Icahn School of Medicine at Mount Sinai, New York City.
To develop a more precise way of identifying women at risk, Li and colleagues turned to artificial intelligence technology to create a “digital phenotype” based on approximately 72,000 births in the Mount Sinai Health System.
The digital tool retrospectively identified about 6600 cases of postpartum hemorrhage, about 3.8 times the roughly 1700 cases that would have been predicted based on methods that estimate blood loss. A blinded physician review of a subset of 45 patient charts — including 26 patients who experienced a hemorrhage, 11 who didn’t, and 6 with unclear outcomes — found that the digital approach was 89% percent accurate at identifying cases, whereas blood loss-based methods were accurate 67% of the time.
Several of the 24 characteristics included in the model appear in other risk predictors, including whether a woman has had a previous cesarean delivery or prior postdelivery bleeding, and whether she has anemia or related blood disorders. Among the rest were risk factors that have been identified in the literature, including maternal blood pressure, time from admission to delivery, and average pulse during hospitalization. Five more features were new: red blood cell count and distribution width, mean corpuscular hemoglobin, absolute neutrophil count, and white blood cell count.
“These [new] values are easily obtainable from standard blood draws in the hospital but are not currently used in clinical practice to estimate postpartum hemorrhage risk,” Li said.
In a related retrospective study, Li and her colleagues used the new tool to classify women into high, low, or medium risk categories. They found that 28% of the women the algorithm classified as high risk experienced a postpartum hemorrhage compared with 15% to 19% of the women classified as high risk by standard predictive tools. They also identified potential “inflection points” where changes in vital signs may suggest a substantial increase in risk. For example, women whose median blood pressure during labor and delivery was above 132 mm Hg had an 11% average increase in their risk for bleeding.
By more precisely identifying women at risk, the new method “could be used to preemptively allocate resources that can ultimately reduce postpartum hemorrhage morbidity and mortality,” Li said. Sema4 is launching a prospective clinical trial to further assess the algorithm, she added.
Finding the Continuum of Risk
Holly Ende, MD, an obstetric anesthesiologist at Vanderbilt University Medical Center, Nashville, Tennessee, said approaches that leverage electronic health records to identify women at risk for hemorrhage have many advantages over currently used tools.
“Machine learning models or statistical models are able to take into account many more risk factors and weigh each of those risk factors based on how much they contribute to risk,” Ende, who was not involved in the new studies, told Medscape. “We can stratify women more on a continuum.”
But digital approaches have potential downsides.
“Machine learning algorithms can be developed in such a way that perpetuates racial bias, and it’s important to be aware of potential biases in coded algorithms,” Li said. To help reduce such bias, they used a database that included a racially and ethnically diverse patient population, but she acknowledged that additional research is needed.
Ende, the co-author of a December commentary in Obstetrics & Gynecology on risk assessment for postpartum hemorrhage, said algorithm developers must be sensitive to preexisting disparities in healthcare that may affect the data they use to build the software.
She pointed to uterine atony — a known risk factor for hemorrhage — as an example. In her own research, she and her colleagues identified women with atony by searching their medical records for medications used to treat the condition. But when they ran their model, Black women were less likely to develop uterine atony, which the team knew wasn’t true in the real world. They traced the problem to an existing disparity in obstetric care: Black women with uterine atony were less likely than women of other races to receive medications for the condition.
“People need to be cognizant as they are developing these types of prediction models and be extremely careful to avoid perpetuating any disparities in care,” Ende cautioned. On the other hand, if carefully developed, these tools might also help reduce disparities in healthcare by standardizing risk stratification and clinical practices, she said.
In addition to independent validation of data-based risk prediction tools, Ende said ensuring that clinicians are properly trained to use these tools is crucial.
“Implementation may be the biggest limitation,” she said.
Ende and Li have disclosed no relevant financial relationships.
J Am Med Inform Assoc. Published in the February 2022 edition.
A comprehensive digital phenotype for postpartum hemorrhage
Improving postpartum hemorrhage risk prediction using longitudinal electronic medical records
Bridget M. Kuehn is a medical writer in Chicago.
For more news, follow Medscape on Facebook, Twitter, Instagram, YouTube, and LinkedIn